News Release 09-054
Maybe Robots Dream of Electric Sheep, But Can They Do Science?
Researchers use algorithm developed for self-repairing robots to uncover scientific laws hidden in raw data
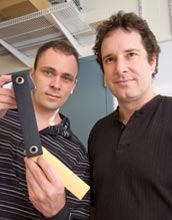
Cornell University researchers Hod Lipson (right) and Michael Schmidt hold up a double pendulum.
April 2, 2009
Listen to a teleconference with Cornell University professor Hod Lipson, doctoral student Michael Schmidt and reporters.
This material is available primarily for archival purposes. Telephone numbers or other contact information may be out of date; please see current contact information at media contacts.
Using the digital mind that guides their self-repairing robot, researchers at Cornell University have created a computer program that uses raw observational data to tease out fundamental physical laws. The breakthrough may aid the discovery of new scientific truths, particularly for biological systems, that have until now eluded detection.
Reporting in the April 3, 2009, issue of Science, Cornell University Mechanical Engineering professor Hod Lipson and his doctoral student Michael Schmidt report that their algorithm can distill fundamental natural laws from mere observations of a swinging double pendulum and other simple systems.
Without any prior instruction about the laws of physics, geometry or kinematics, the algorithm driving the computer's number crunching was able to determine that the swinging, bouncing and oscillating of the devices arose from specific fundamental processes.
The algorithm deciphered in hours the same Laws of Motion and other properties that took Isaac Newton and his successors centuries to realize.
The new breakthrough is not far removed from Lipson's earlier NSF CAREER award work to develop Starfish, a robot with a "self-image" that could repair itself when damaged.
"The way the robot managed to recover from damage was to create a dynamical model, a self-image," said Lipson. "It then used that model to make predictions about itself."
A dynamical model is a mathematical representation of the way in which a system's components influence each other over time. Lipson and Schmidt realized that if a robot can create dynamical models from data about itself, why not attempt to model the surrounding world as well?
When Lipson and Schmidt experimented with that approach, they learned their algorithm was re-discovering laws that were well known to scientists and engineers, suggesting the algorithm should be able to help uncover new laws for data sets that are less well understood.
"What is fascinating is that in the same way a robot created a dynamical model of itself using robot pieces, we now can create models not from motors and joints, but from components of mathematical objects, like variables, symbols like + and -, and other mathematical operators and functions," said Lipson.
While the algorithm can work with almost any data set, for this experiment Lipson and Schmidt used motion-capture data of pendulums and oscillators--similar to the motion capture techniques used for movie special-effects. The researchers then fed the data to a computer running the new algorithm, a process modeled on the one driving their Starfish robot.
The computer began its analysis with a broad suite of mathematical building blocks, expressions that the computer could combine to recreate patterns in the data set. Using a computational process called symbolic regression, a process inspired by biological evolution, the computer then took the assemblage of expressions and competed them against each other to find matches that reflected the data. The goal was to find those aspects of the data that were invariant, that did not change from one observation to the next.
"When you look at a pendulum, for example, some things go up, some go down," said Lipson. "But to recognize that when something goes up another specific thing always go down to keep the total sum constant, this is a key to understanding the observations in a deeper sense--such as recognizing the laws of conservation."
The computer retained the mathematical expressions that were invariant and abandoned those that were not, leaving a set of expressions that matched the data set and predicted future behavior. Because such a process could find patterns that are merely coincidental, the new algorithm also contains a critical step that compares subcomponent expressions, evaluating invariant equations to show that they are meaningful and represent actual natural laws, proof that the results are truly predictive.
Ultimately, a human still has to take the final list of a dozen or so expressions and figure out what they reflect in reality--for example, which expressions are describing a motion or energy-conservation law, or something totally new. Humans are still critical to the process: the computer serves as a data miner to find the laws, but a human must interpret them and give them meaning.
"Physicists like Newton and Kepler could have used a computer running this algorithm to figure out the laws that explain a falling apple or the motion of the planets with just a few hours of computation," said Schmidt, "but a human still needs to pick the appropriate building-blocks and framework, as well as give words and interpretation to laws found by the computer."
In the future, Lipson and Schmidt plan to use the new approach for biological systems. Biology is notoriously complicated to model, and finding fundamental laws for such systems can be difficult. With the new algorithm, the enormous data sets researchers collect about biological systems may yield invariants, unchanging aspects that may reveal underlying fundamental laws.
For this study, Michael Schmidt was supported by an NSF GRFP fellowship. Hod Lipson was supported by NSF CAREER Award 0547376 and NSF Creative-IT grant 0757478.
-NSF-
-
Play Audio
Cornell researcher Hod Lipson and his doctoral student Michael Schmidt discuss their findings.
Credit and Larger Version -
The double pendulum used by Cornell researchers Hod Lipson and Michael Schmidt.
Credit and Larger Version -
The airtrack used by Cornell researchers Hod Lipson and Michael Schmidt.
Credit and Larger Version -
Cornell University doctoral student Michael Schmidt sets up testing equipment.
Credit and Larger Version -
The researchers' findings were published in the April 3, 2009, issue of Science magazine.
Credit and Larger Version
Media Contacts
Joshua A. Chamot, NSF, (703) 292-7730, email: jchamot@nsf.gov
Blaine Friedlander, Cornell University, (607) 254-8093, email: bpf2@cornell.edu
Program Contacts
Mary Lou Maher, NSF, (703) 292-8930, email: mmaher@nsf.gov
Christina L. Bloebaum, NSF, (703) 292-8611, email: cbloebau@nsf.gov
Principal Investigators
Hod Lipson, Cornell University, (607) 255 1686, email: HL274@cornell.edu
Co-Investigators
Michael Schmidt, Cornell University, (607) 254 8940, email: mds47@cornell.edu
Related Websites
Researchers Wanted: Humans Need Not Apply?: /news/news_summ.jsp?cntn_id=114512&org=NSF&from=news
The U.S. National Science Foundation propels the nation forward by advancing fundamental research in all fields of science and engineering. NSF supports research and people by providing facilities, instruments and funding to support their ingenuity and sustain the U.S. as a global leader in research and innovation. With a fiscal year 2023 budget of $9.5 billion, NSF funds reach all 50 states through grants to nearly 2,000 colleges, universities and institutions. Each year, NSF receives more than 40,000 competitive proposals and makes about 11,000 new awards. Those awards include support for cooperative research with industry, Arctic and Antarctic research and operations, and U.S. participation in international scientific efforts.
Connect with us online
NSF website: nsf.gov
NSF News: nsf.gov/news
For News Media: nsf.gov/news/newsroom
Statistics: nsf.gov/statistics/
Awards database: nsf.gov/awardsearch/
Follow us on social
Twitter: twitter.com/NSF
Facebook: facebook.com/US.NSF
Instagram: instagram.com/nsfgov